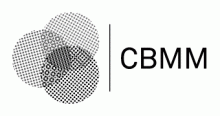
H. N. Mhaskar - Claremont Graduate University, Claremont.
The fundamental problem of machine learning is often formulated as the problem of function approximation. For example, we have data of the form {(xj,yj)}, where yj is the class label for xj, and we want to approximate the class label as a function of the input x. The standard way for this approximation is to minimize a loss functional, usually with some regularization. Surprisingly, even though the problem is posed as a problem of function approxi- mation, approximation theory has played only a marginal role in this theory. We describe our efforts to explore why this might be the case, and also to develop approximation theory/harmonic analysis tools more meaningfully and directly applicable to machine learning. We also argue that classification problems are better treated as generalized signal separation problems rather than function approximation problems.